Selected Publications
Author of over 300 papers with the ATLAS Collaboration. This is a list of selected publications with substantial personal contribution.
Machine Learning Conference Publications
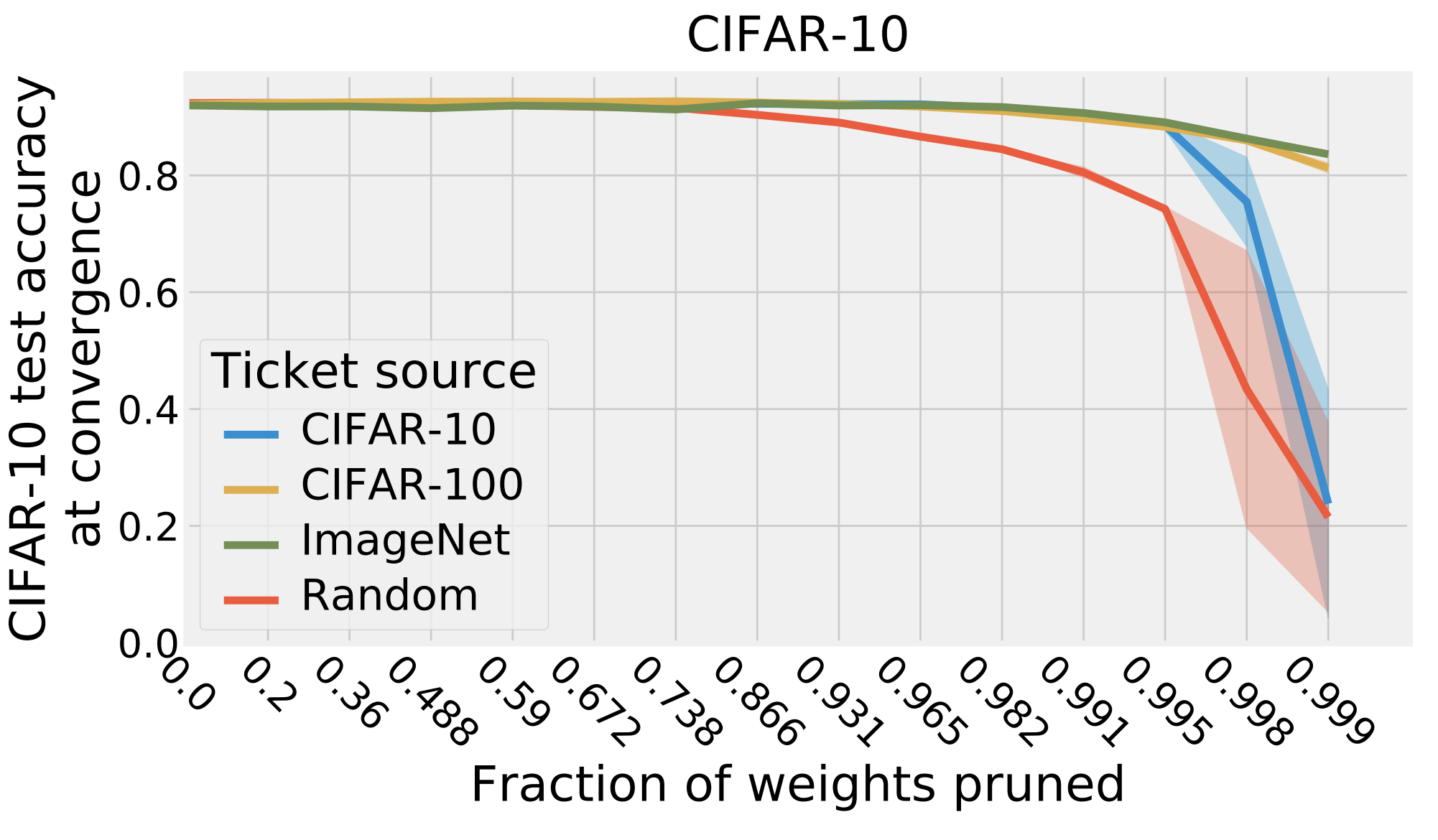
One Ticket to Win Them All: Generalizing Lottery Ticket Initializations across Datasets and Optimizers, A. S. Morcos, H. Yu, M. Paganini, Y. Tian, NeurIPS 2019.
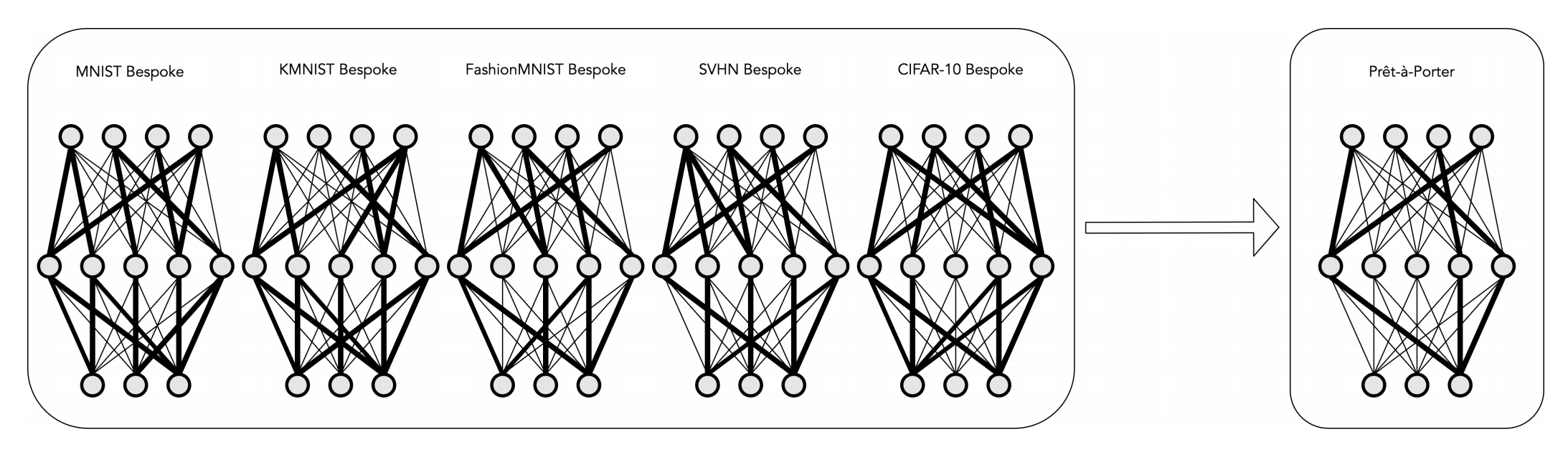
Bespoke vs. Prêt-à-Porter Lottery Tickets: Exploiting Mask Similarity for Trainable Sub-Network Finding, M. Paganini, J. Forde, under review.
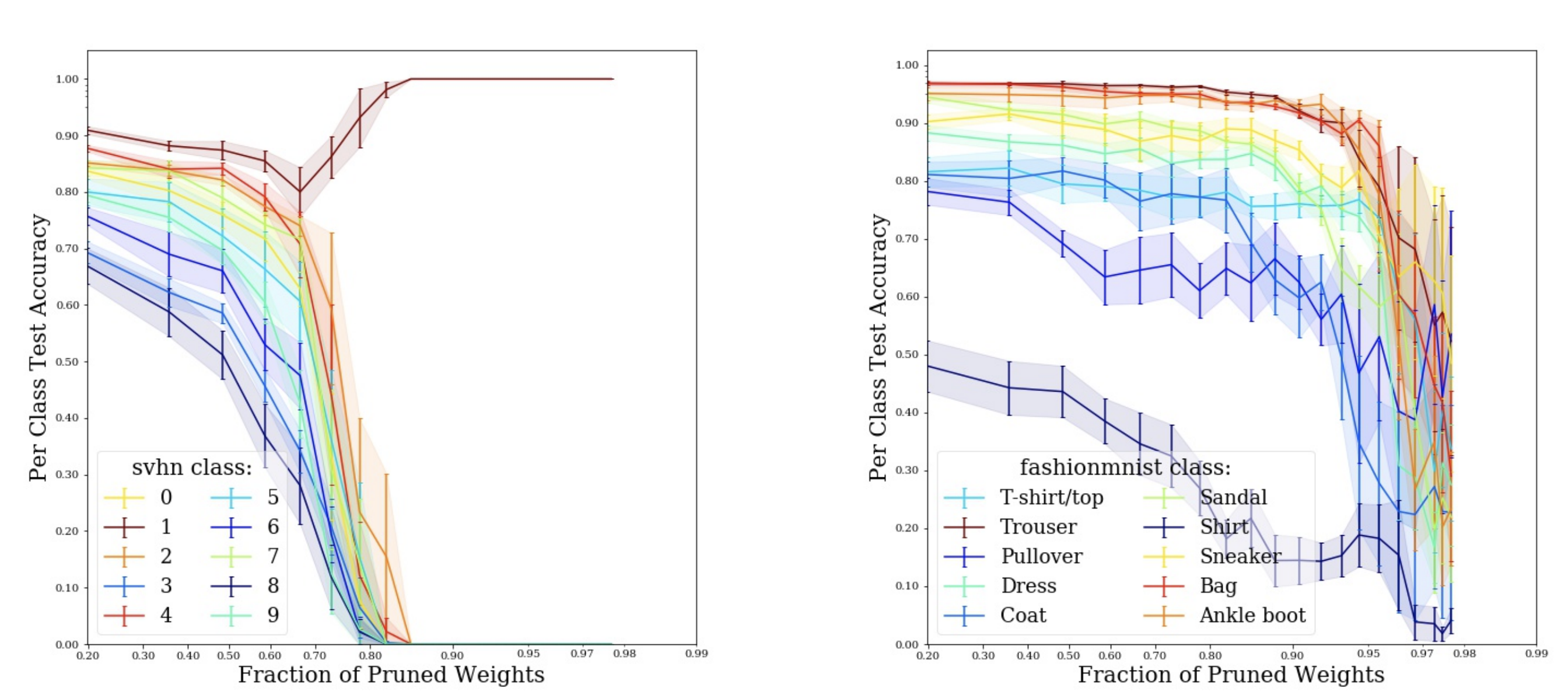
Prune Responsibly, M. Paganini, under review.
Machine Learning Workshop Contributions
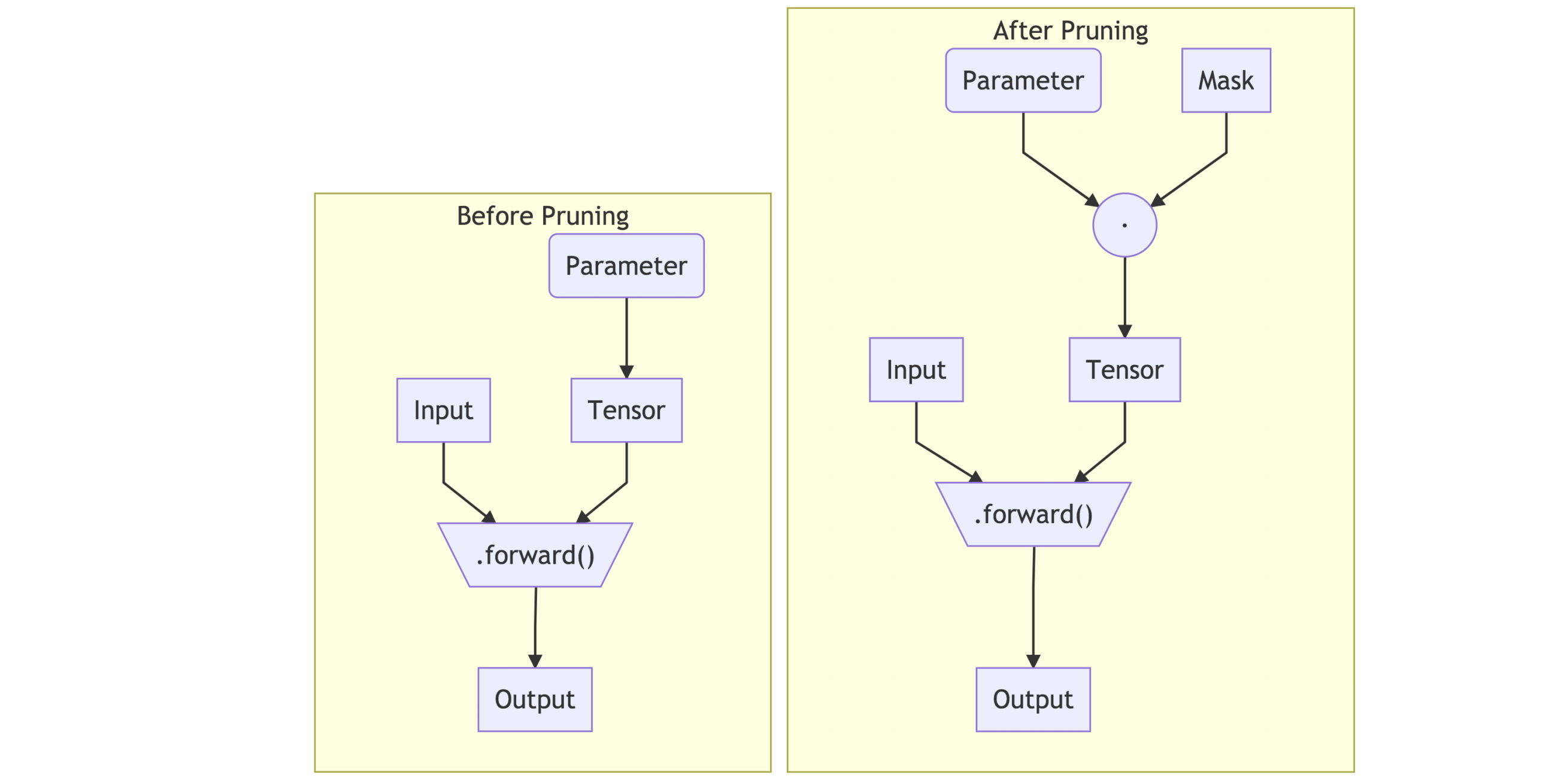
Streamlining Tensor and Network Pruning in PyTorch, M.Paganini, J.Forde, ICLR 2020 "Practical ML for Developing Countries" workshop.
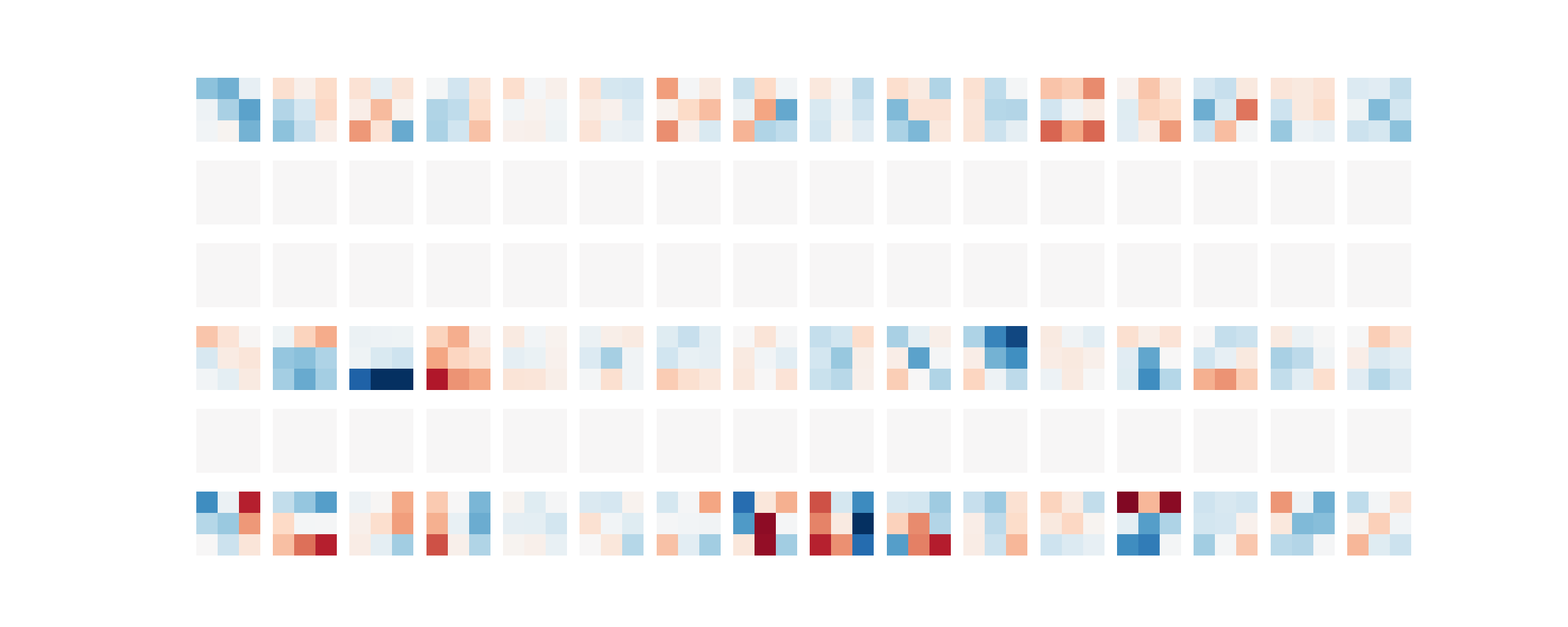
On Iterative Neural Network Pruning, Reinitialization, and the Similarity of Masks, M. Paganini, J. Forde, ICLR 2020 "Practical ML for Developing Countries" workshop.
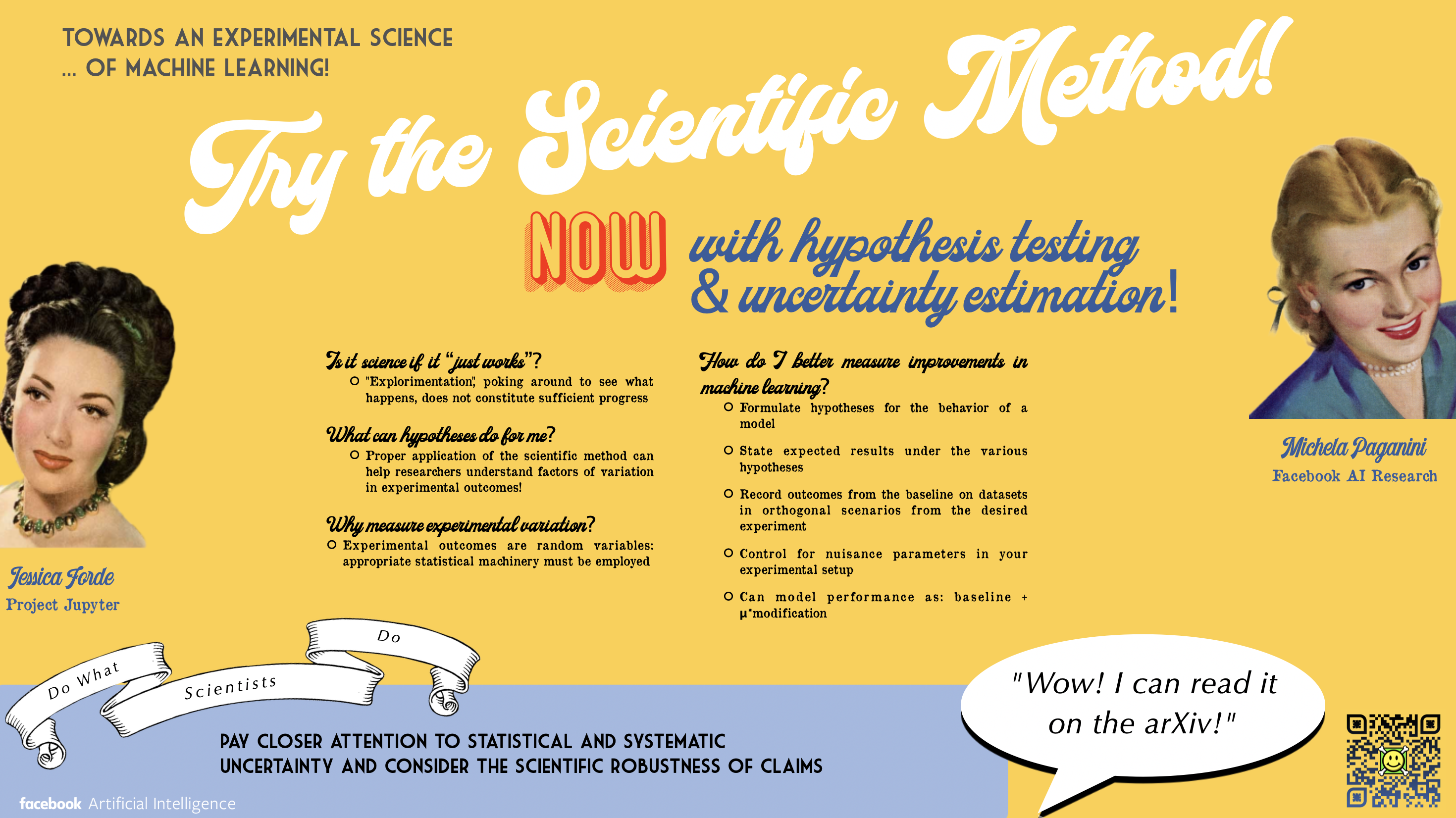
The Scientific Method in the Science of Machine Learning, J. Forde, M. Paganini, ICLR 2019 "Debugging ML" workshop.
Physics Journal Publications
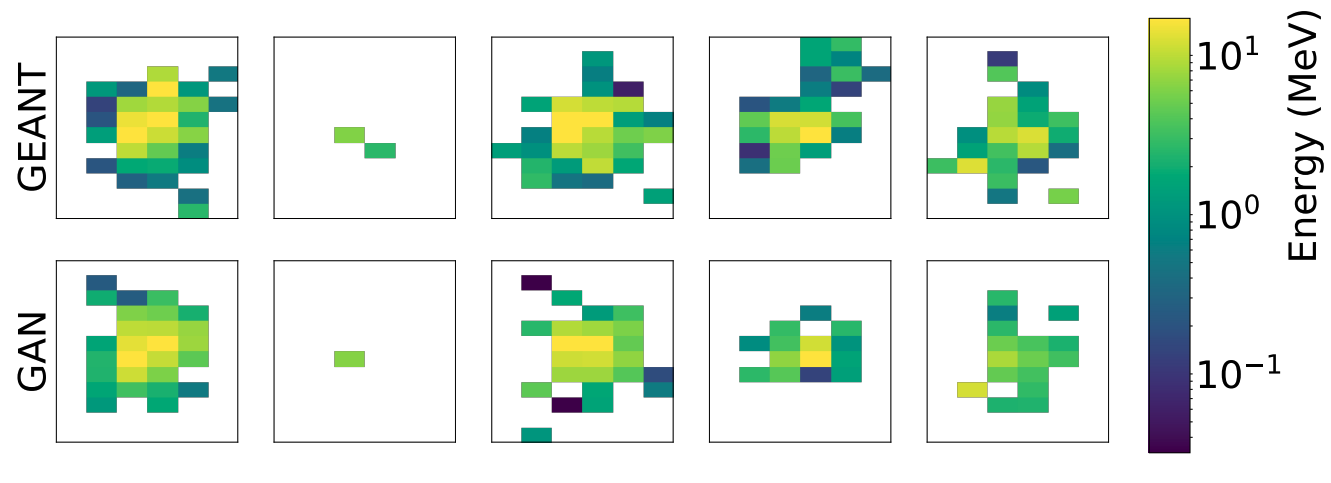
Accelerating Science with Generative Adversarial Networks: An Application to 3D Particle Showers in Multi-Layer Calorimeters, M. Paganini, L. de Oliveira, B. Nachman, Phys. Rev. Lett.
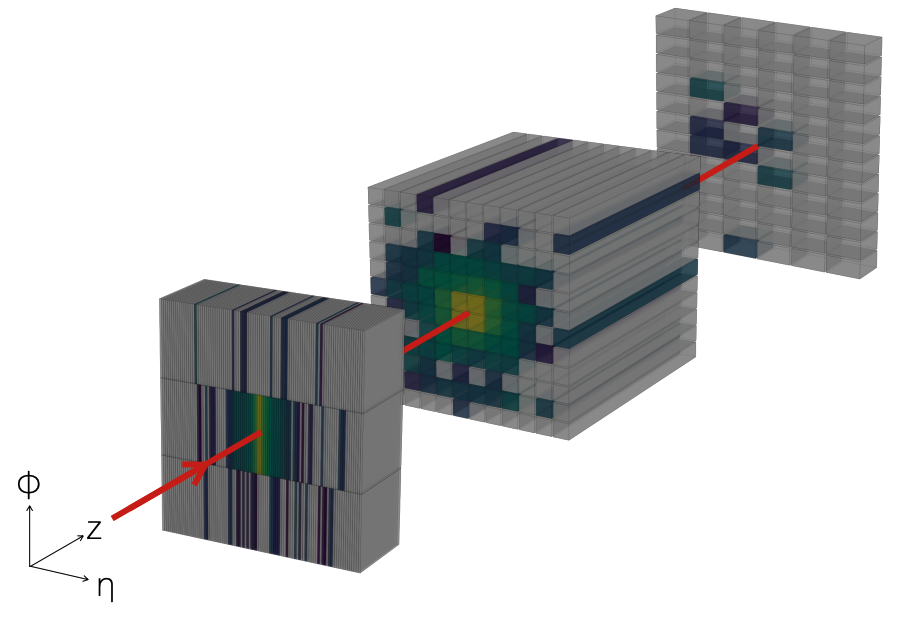
CaloGAN: Simulating 3D High Energy Particle Showers in Multi-Layer Electromagnetic Calorimeters with Generative Adversarial Networks, M. Paganini, L. de Oliveira, B. Nachman, Phys. Rev. D
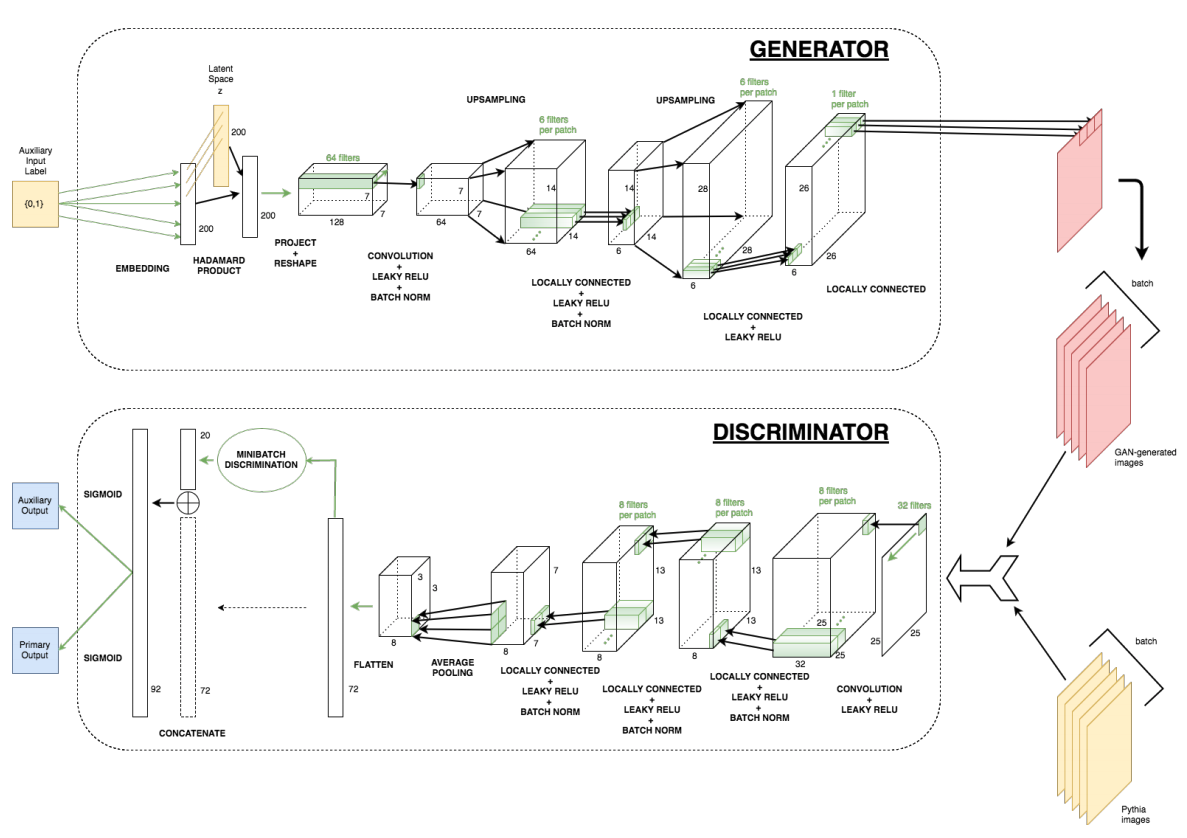
Learning Particle Physics by Example: Location-Aware Generative Adversarial Networks for Physics Synthesis, L. de Oliveira, M. Paganini, B. Nachman, Comput Softw Big Sci.
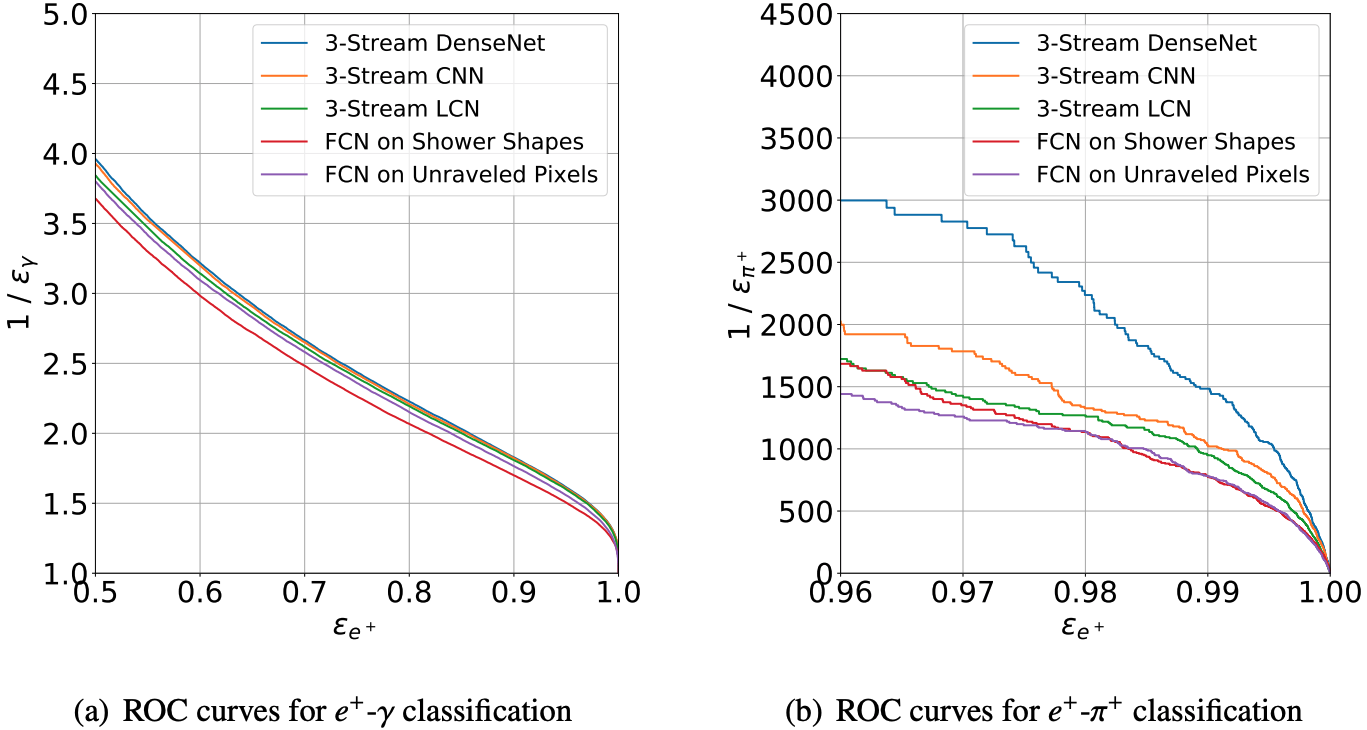
Electromagnetic Showers Beyond Shower Shapes, L. de Oliveira, B. Nachman, M. Paganini, NIMA.
Physics Conference Publications
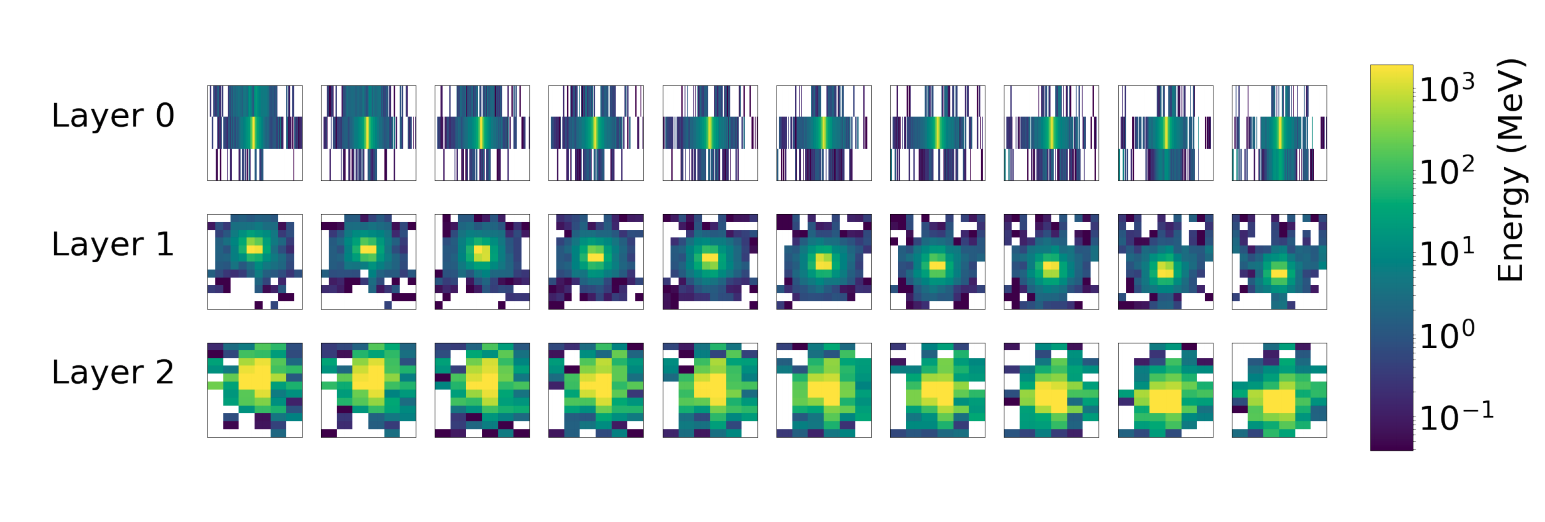
Controlling Physical Attributes in GAN- Accelerated Simulation of Electromagnetic Calorimeters, M. Paganini, L. de Oliveira, B. Nachman, in Proceedings of ACAT 2017.
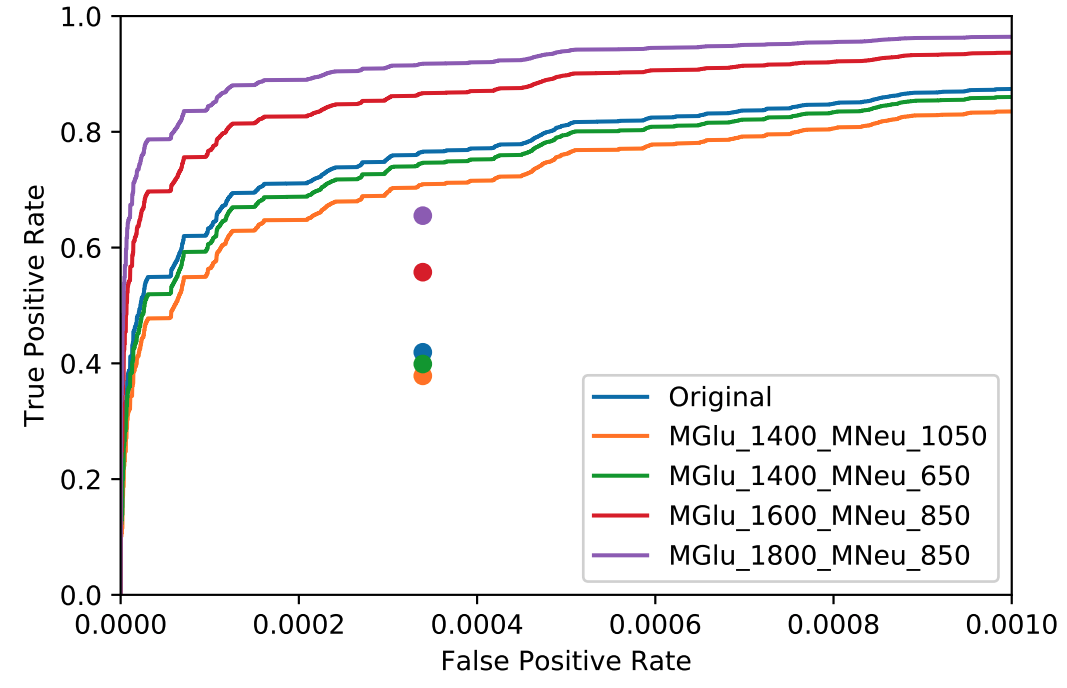
Neural Networks for Physics Analysis on low-level whole-detector data at the LHC, W. Bhimji et al., in Proceedings of ACAT 2017.
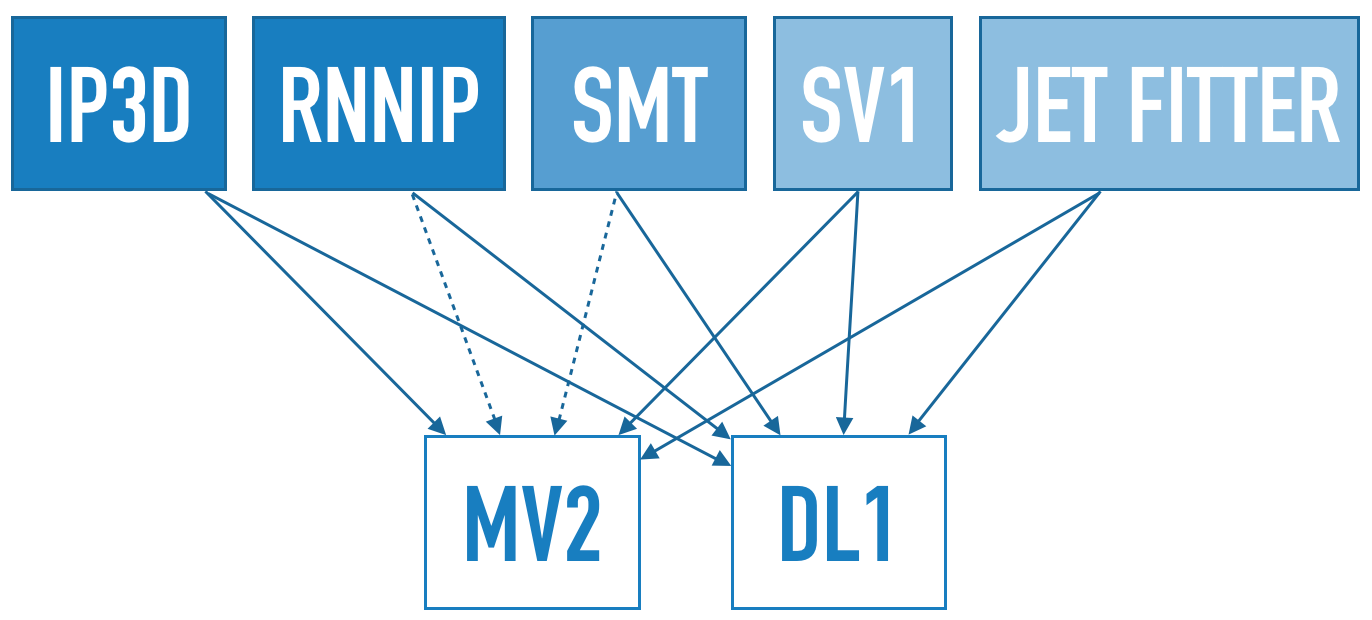
Machine Learning Algorithms for b-jet tagging at the ATLAS experiment, M. Paganini, in Proceedings of ACAT 2017.
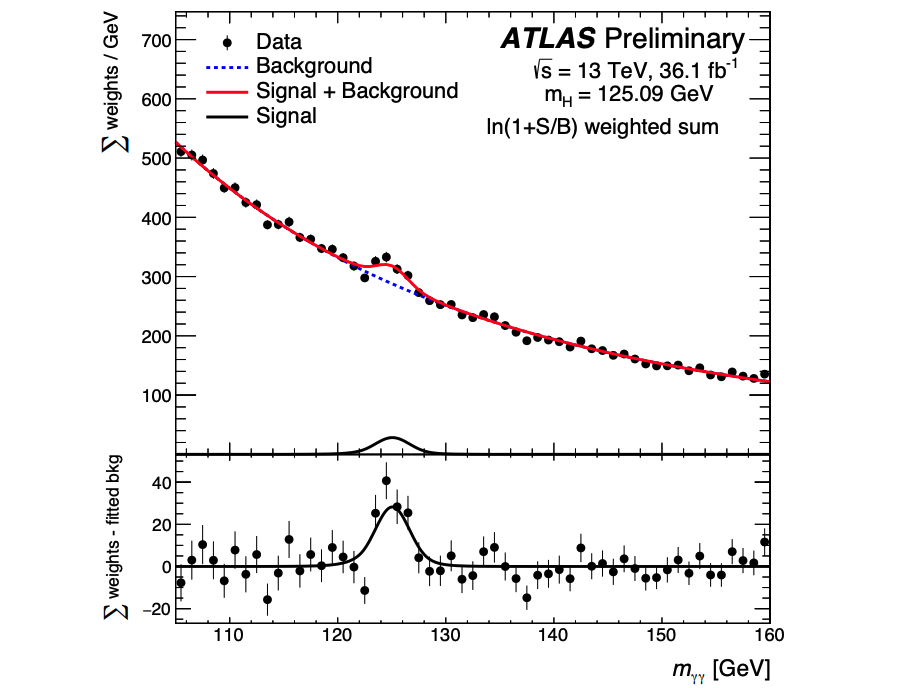
Measurements of Higgs boson properties in the diphoton decay channel with 36.1 fb$^{−1}$ pp collision data at the center-of-mass energy of 13 TeV with the ATLAS detector, The ATLAS Collaboration, in Proceedings of EPS-HEP 2017.
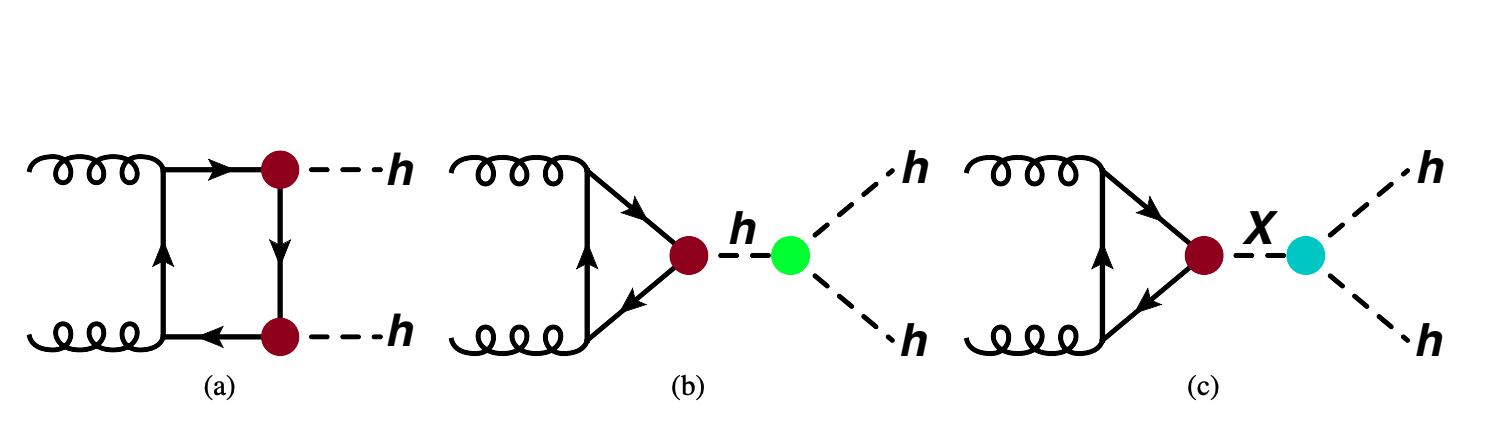
Search for Higgs boson pair production in the $bb\gamma\gamma$ final state using $pp$ collision data at $\sqrt{s}$= 13 TeV with the ATLAS detector, The ATLAS Collaboration, in Proceedings of the 50th Rencontres de Moriond EW.
ATLAS PUB Notes
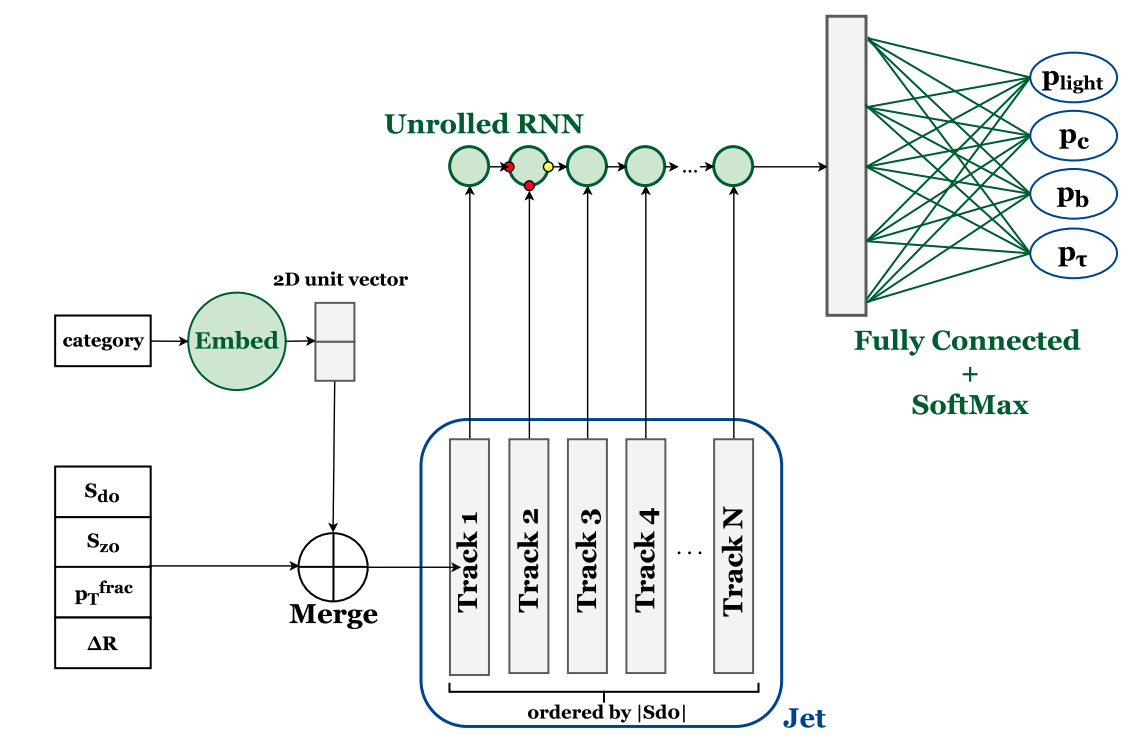
Identification of Jets Containing $b$-Hadrons with Recurrent Neural Networks at the ATLAS Experiment, The ATLAS Collaboration.
PhD Thesis
Pre-Prints
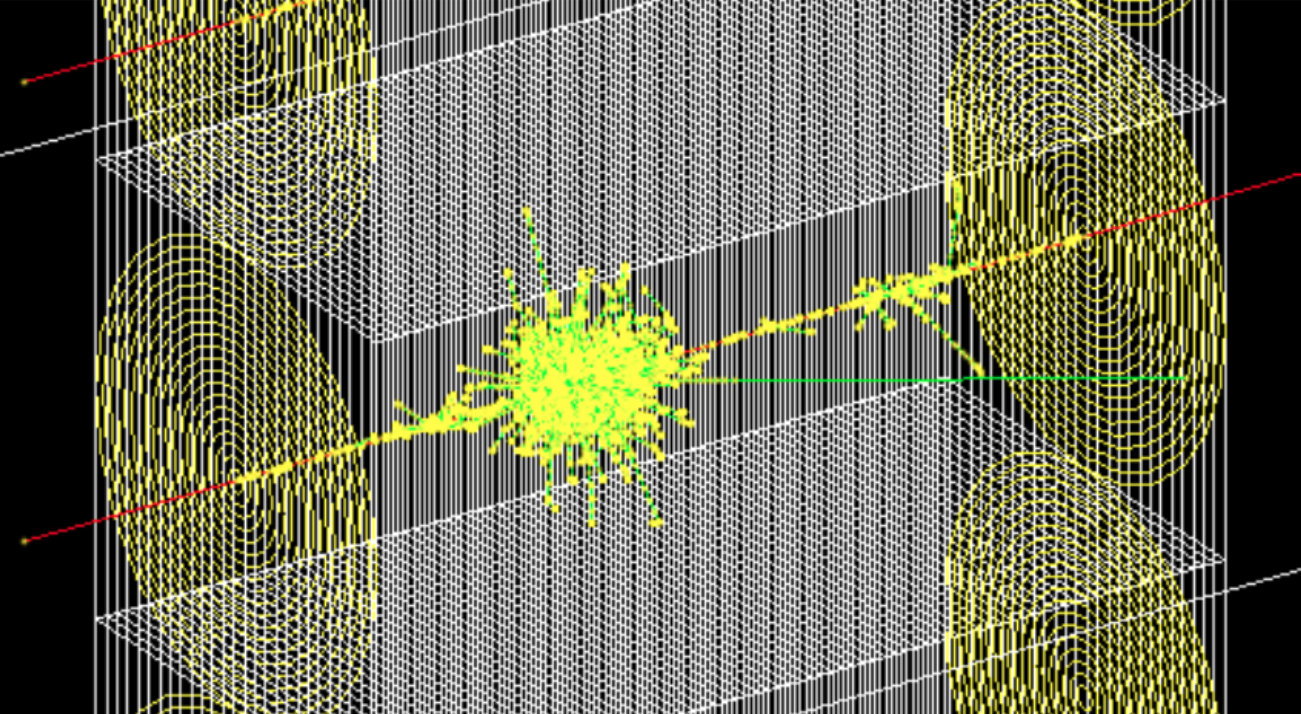
HEP Software Foundation Community White Paper Working Group - Detector Simulation, HEP Software Foundation: J. Apostolakis et al.
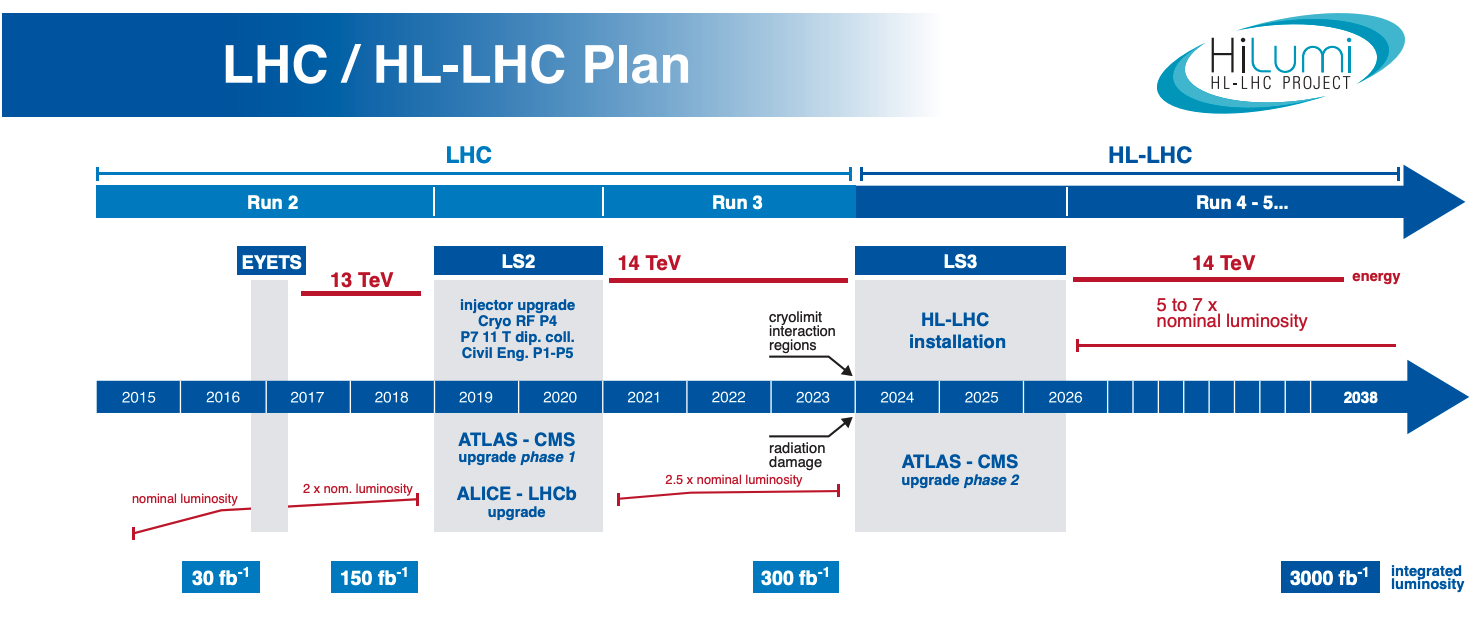
A Roadmap for HEP Software and Computing R&D for the 2020s, HEP Software Foundation: J. Albrecht et al.